Large-Scale Simulation Technology Using Mechanical Learning Potential
We have developed a machine learning algorithm that identifies interatomic potentials using the results of ab initio calculations as training data. This algorithm enables us to predict the catalytic activity of nanoparticle surfaces (up to 3,000 atoms) and the lithium (Li) diffusion behavior accompanying the structural phase transition of solid Li ion conductors.
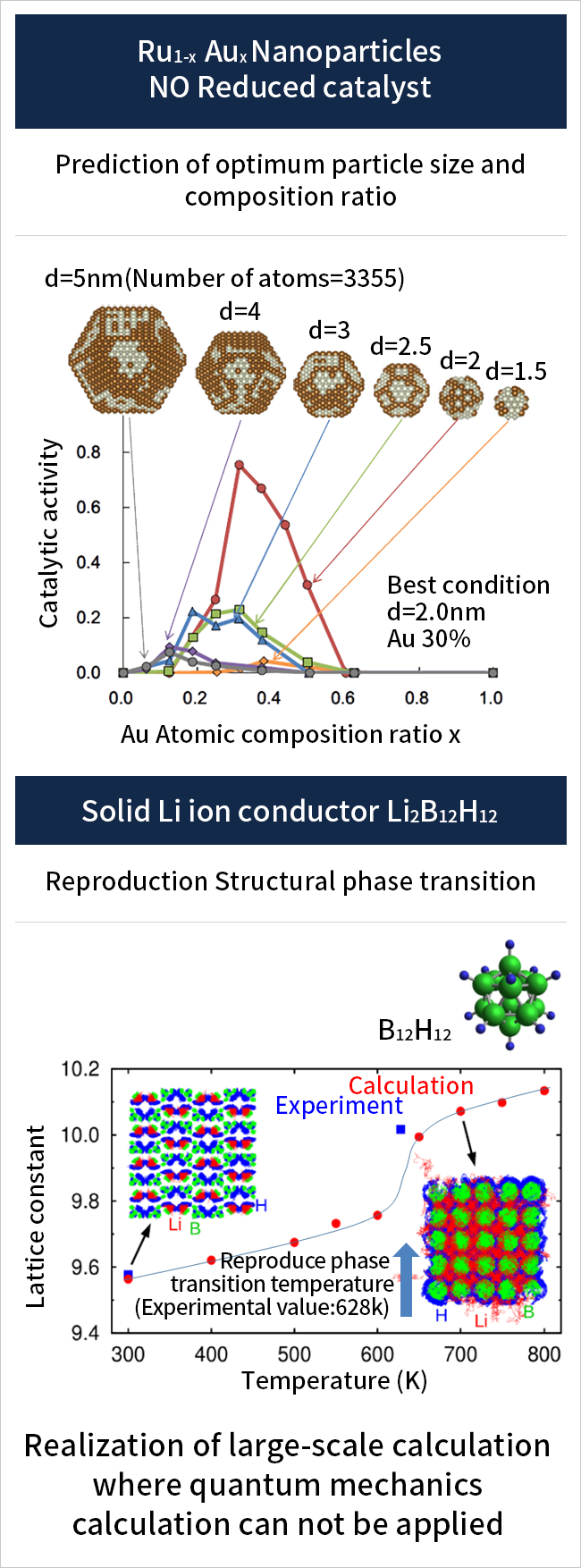